AI in Engineering: Turning Complex Data into Better Decisions
- SIG ML
- Feb 3
- 5 min read
Updated: Mar 6
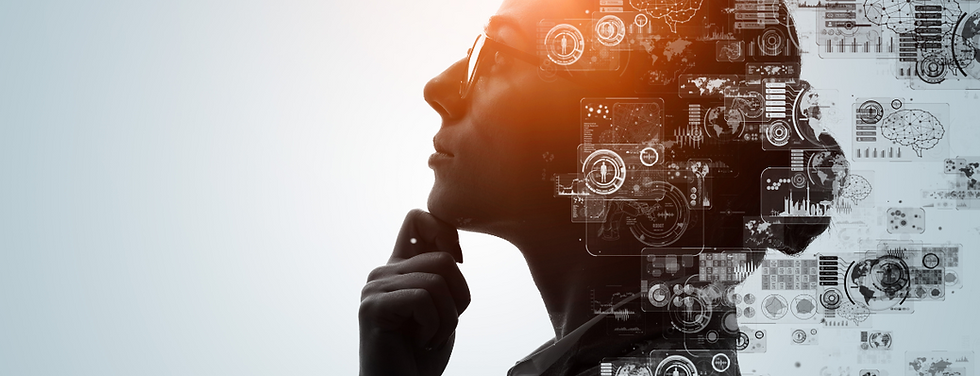
Engineering in a Data-Driven World
Engineering decisions rely on experience, but in operational settings, where engineers work with real-world systems and processes, the challenge is making sense of increasing volumes of data to improve efficiency and reliability.
Operating assets and systems, from energy and mining to motorsports, generate vast amounts of sensor data. Turning this data into meaningful insights that lead to better decisions is not always straightforward. Engineers need reliable information, but manually reviewing data takes time, and traditional models often rely on assumptions that don’t always reflect real-world conditions.
Artificial Intelligence (AI) and machine learning (ML) are now being used to help engineers process and interpret data more efficiently. These models analyse information from sensors, as well as simulations and other operational datasets, to recognise patterns that might otherwise go unnoticed. By integrating AI into engineering workflows, teams can make faster, more informed decisions with a clearer understanding of where to focus their efforts. Rather than relying solely on experience to determine what to prioritise—often under time constraints—AI provides a structured way to surface important insights. Engineers can apply their expertise where it adds the most value, ensuring that decisions are made with a full view of the system rather than only addressing the most apparent issues.
Challenges in Engineering Decision-Making
Modern engineering environments generate large volumes of data, yet using all this data effectively remains a challenge. Engineers work with increasingly complex systems where decisions must balance performance, cost, reliability, and sustainability. The opportunity to optimise operations is there, but several barriers make it difficult to extract value from various data sources available.
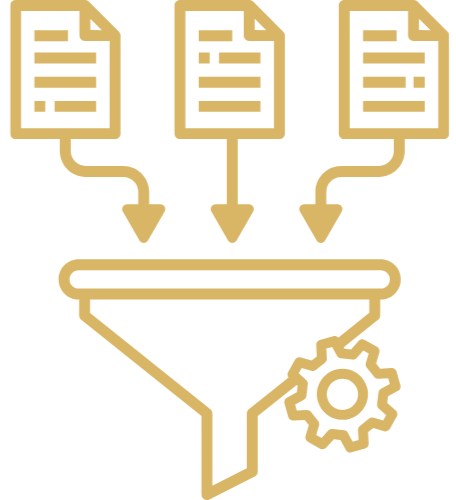
One of the main challenges is data overload. Sensors, control systems, and historical records provide more information than engineers can realistically review. With limited time and resources, decisions are often made based on what stands out rather than a complete understanding of the system.
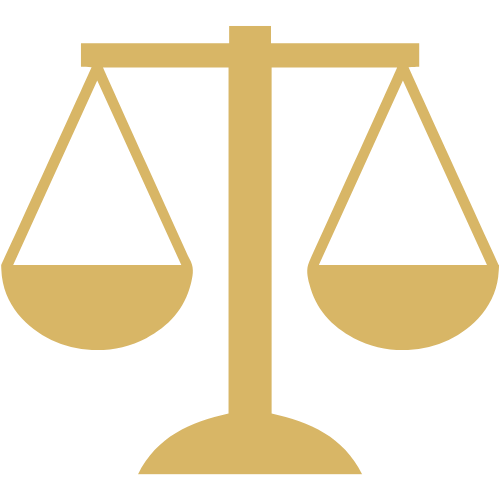
Another key challenge is managing competing priorities. Engineers must make trade-offs between efficiency, performance, and reliability, but in interconnected systems, adjusting one variable can have unintended consequences elsewhere. Without the ability to test different approaches quickly, optimising across multiple constraints becomes increasingly difficult.
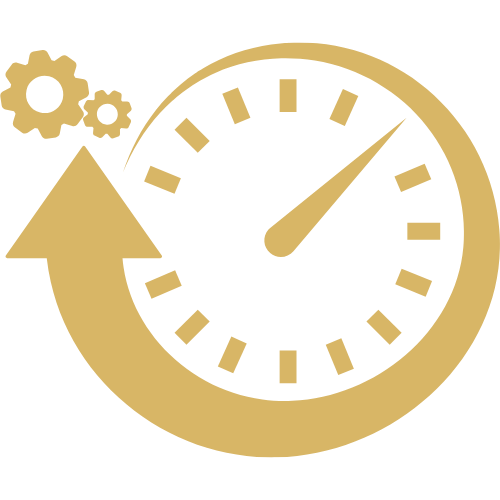
In many cases, time and resource constraints prevent deeper analysis. Engineering teams can operate under tight schedules, leaving little room to explore alternative strategies or experiment with different configurations. As a result, decisions are often made based on experience, partial data, or simplified models, missing opportunities for greater efficiency.
These challenges are not new, but with the increasing scale and complexity of modern systems, they are becoming harder to manage.
How AI is Enhancing Engineering Decision-Making
AI offers a way to support engineers by making it easier to process large amounts of data, test different scenarios, and ensure that decisions align with operational priorities. AI does not replace engineering expertise—it enhances it by providing better visibility into system behaviours, identifying patterns in large datasets, and reducing the time needed to analyse operational conditions. Rather than manually searching for key insights, engineers can use AI to surface meaningful trends and test different approaches before implementing changes.
One way AI improves decision-making is by unifying data across assets and systems, building models that learn by example how to form a connected view of operations. Instead of working with siloed datasets, engineers can access a broader and generalised perspective, allowing them to detect trends and dependencies that might otherwise go unnoticed.
AI also supports predictive modelling, helping engineers anticipate potential issues or inefficiencies before they occur. By analysing historical and real-time data, models can highlight patterns that indicate emerging issues, allowing engineers to take proactive steps rather than reacting to unexpected disruptions.
Another advantage is the ability to run fast simulations and optimisations. Engineers can test multiple scenarios under different constraints, comparing potential strategies in a way that would not be feasible with traditional modelling approaches. This enables teams to evaluate different trade-offs and adjust their approach based on real-world data rather than assumptions alone.
By integrating AI into engineering workflows, teams can focus on applying their expertise to the most critical challenges. AI does not change how decisions are made—it improves the speed, accuracy, and confidence with which engineers can make them.
Bringing AI into Engineering Workflows
For AI to be effective within engineering domains, it must integrate seamlessly into existing tools, processes and ways of working. Engineers work with complex systems that have been refined over time, and AI should enhance these workflows rather than disrupt them. The value of AI comes from guiding decision-making in a way that is transparent, reliable, and grounded in engineering principles.
One of the key challenges in AI adoption is trust. Engineers rely on well-understood models and methodologies, and any new approach must be tested and validated before it becomes part of critical decision-making. AI models should not be seen as black boxes but as assistive tools that provide structured guidance based on real-world data. When built correctly, AI-driven insights reflect physical realities and operational constraints, making them a natural extension of engineering analysis rather than a replacement for it.
Another factor in effective AI adoption is adaptability. Engineering environments are dynamic, with changing conditions and evolving requirements. AI systems must be able to learn from new data and adjust to different scenarios without requiring extensive reconfiguration. Models that continuously refine themselves based on operational feedback can provide more accurate, context-aware recommendations that engineers can trust.
When AI is embedded properly, engineers can focus on decision-making and strategy rather than manual data analysis. Instead of spending time filtering through vast amounts of information, they can use AI-generated insights to assess different options, test strategies, and implement solutions with greater confidence.
How SIG Machine Learning Supports AI Integration
At SIG Machine Learning we empower engineers to make better use of their data by providing the applications and tools they need to move from traditional software to advanced AI-driven modelling and optimisation. Our Nexgineer™ platform is designed to seamlessly integrate AI into engineering workflows, helping teams eliminate time-consuming, assumption-heavy processes.
Nexgineer™ enables teams to:
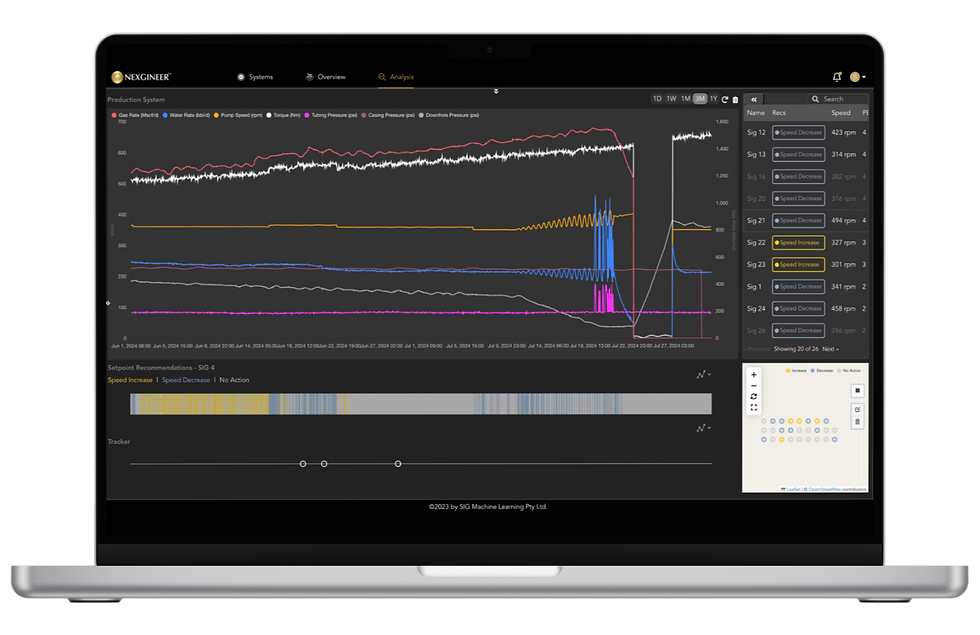
Extract insights from large datasets without requiring manual review.
Run simulations and optimisations to test different strategies under real-world constraints.
Predict operational trends using models that continuously improve over time.
Integrate AI into existing systems without disrupting established workflows.
Next Steps
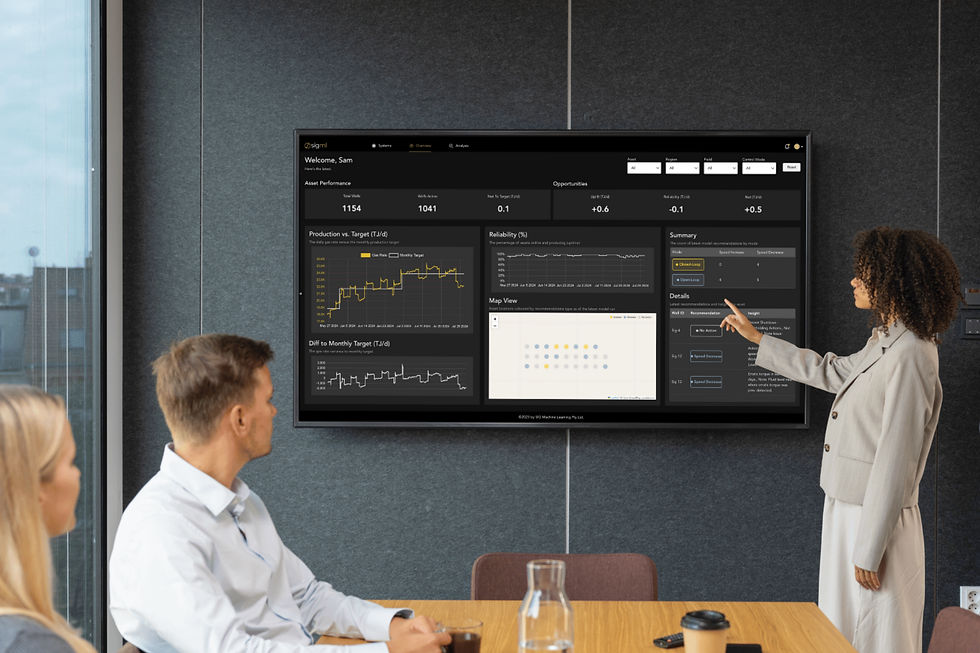
AI is already transforming how engineers work with data, but its impact depends on how well it is integrated into decision-making. Whether your team is exploring AI for the first time or scaling its use across operations, we have experience deploying AI in solutions ranging from advanced surveillance to intelligent control systems. The key is ensuring that AI remains an engineering tool—one that supports expertise rather than replacing it.
If you're looking to explore how AI can enhance your engineering and operations workflows, we'd be happy to discuss its applications for your specific challenges. For a demo, industry use cases, or implementation plans, contact us or email: hello@sigmachinelearning.com